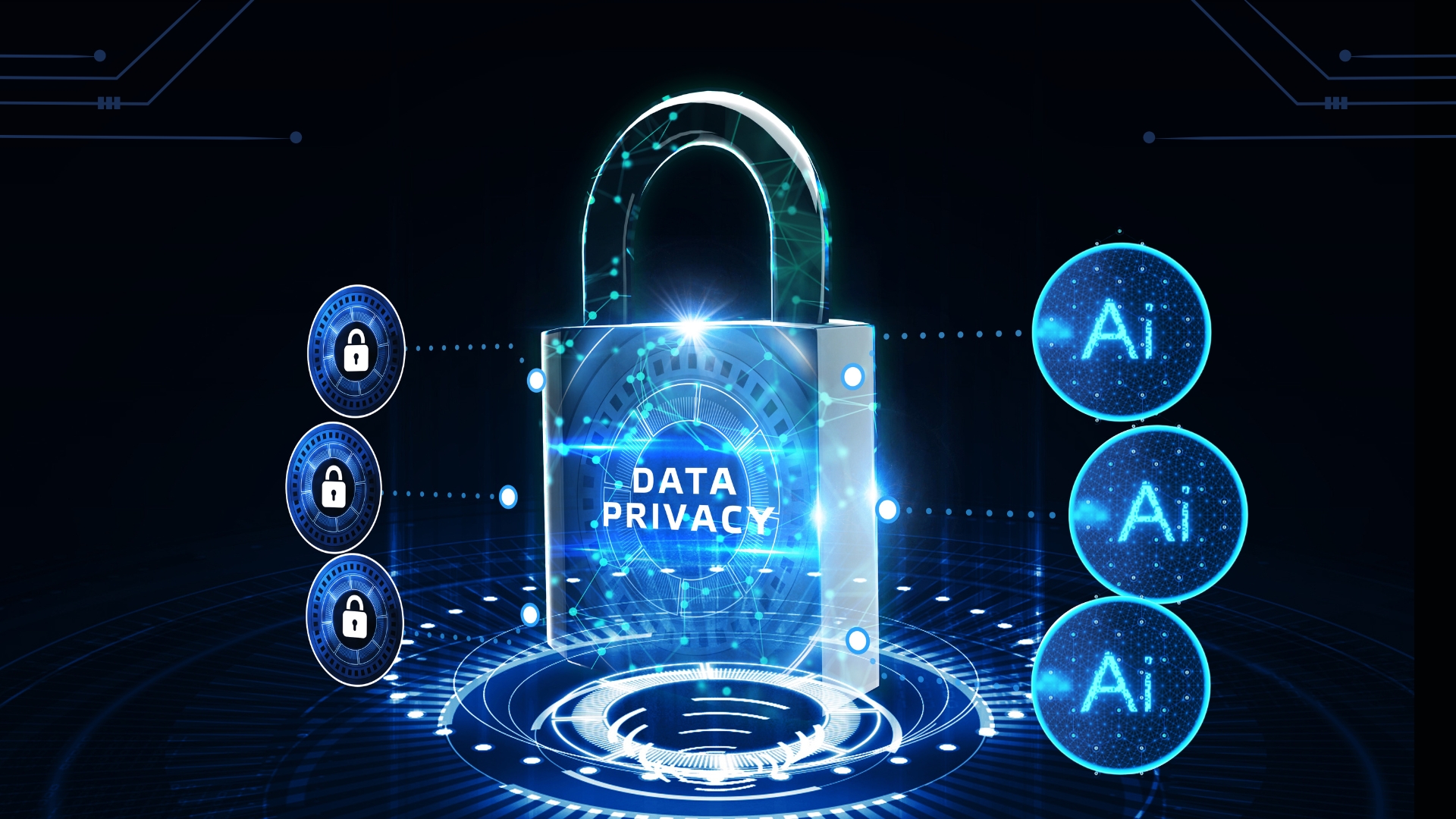
In today’s rapidly evolving technological landscape, autonomous industrial AI systems are becoming increasingly prevalent. These systems have the ability to revolutionize industries, promote creativity, and raise productivity. But immense power also entails great responsibility.
We must address the ethical issues of prejudice and data privacy as we continue to integrate AI into industrial applications. We will delve into these topics in-depth in this essay, highlighting the significance of implementing ethical AI in industrial contexts.
Introduction
The advent of autonomous industrial AI has brought about significant advancements in various sectors, from manufacturing to logistics. These AI systems can complete difficult jobs with little assistance from humans since they are driven by machine learning algorithms. Even while there is no denying the advantages, responsible AI deployment requires addressing some urgent ethical issues.
One of the primary ethical considerations in autonomous industrial AI is bias. Bias in AI can lead to unfair outcomes, discrimination, and unintended consequences. Furthermore, because AI systems frequently require enormous volumes of data in order to operate efficiently, data privacy is an important concern. Ensuring that this data is handled securely and ethically is paramount.
The various forms of bias that might impact industrial AI will be examined in this essay, along with the significance of data privacy and best practices for minimizing bias and boosting data security. Understanding these ethical considerations can pave the way for responsible AI implementation that benefits all stakeholders.
Bias in Autonomous Industrial AI
Bias in AI refers to systematic errors that result in unfair or prejudiced outcomes. In the context of autonomous industrial AI, bias can manifest in various ways, impacting decision-making processes and overall system performance. Addressing bias is crucial to ensure that AI systems operate fairly and equitably.
Types of Bias in Industrial AI
- Algorithmic Bias: This occurs when the algorithms themselves are biased. It can stem from the way the algorithms are designed or the assumptions made during their development. For example, an AI system designed to predict equipment failures may be biased towards certain types of machinery, leading to inaccurate predictions for others.
- Data Bias: Data is the lifeblood of AI systems. If the data used to train AI models is biased, the resulting models will also be biased. Data bias can occur due to historical inequalities, sampling errors, or unrepresentative data. For instance, if an industrial AI system is trained on data from predominantly male workers, it may not perform as well for female workers.
- Interaction Bias: Interaction bias arises from the way users interact with AI systems. Over time, user behavior can influence the AI’s outputs, leading to biased results. For example, if operators consistently provide positive feedback for certain types of automated decisions, the AI system may become biased toward those decisions.
Data Privacy in Autonomous Industrial AI
Data privacy is a fundamental ethical consideration in the development and deployment of autonomous industrial AI systems. Access to enormous volumes of data, particularly private and sensitive information, is frequently necessary for these systems. Ensuring responsible use and preservation of this data is essential to maintaining integrity and confidence.
Importance of Data Privacy
- Protecting Sensitive Information: Sensitive data, including personnel records, operational specifics, and trade secrets, may be handled by industrial AI systems. Unauthorized access to private data can have serious repercussions, such as harm to one’s reputation and financial loss.
- Compliance with Regulations: Various regulations, such as the General Data Protection Regulation (GDPR), mandate strict data privacy measures. Serious fines and legal problems may follow noncompliance. Ensuring that AI systems adhere to these regulations is crucial for ethical AI implementation.
- Building Trust: Establishing trust with stakeholders, such as partners, consumers, and employees, depends on data privacy. When stakeholders trust that their data is being handled responsibly, they are more likely to support and adopt AI technologies.
Best Practices to Reduce AI Bias and Improve Data Security
Addressing bias and ensuring data privacy in autonomous industrial AI systems requires a proactive and comprehensive approach.
Reducing AI Bias
- Diverse Data Collection: Ensure that the data used to train AI models is diverse and representative of the entire population. This helps mitigate data bias and improves the generalizability of the models.
- Bias Audits: Regularly conduct bias audits to identify and address any biases in the AI system. These audits should include a thorough examination of the algorithms, data, and outputs.
- Transparent Algorithms: Develop transparent algorithms that provide insights into how decisions are made. Transparency helps identify and address biases and build trust among users.
- Inclusive Development Teams: Assemble diverse and inclusive development teams to bring different perspectives and reduce the risk of bias in AI systems.
Improving Data Security
- Data Encryption: Implement robust encryption methods to protect data at rest and in transit. Encryption ensures that sensitive information remains secure even if unauthorized access occurs.
- Access Controls: Establish strict access controls to limit who can access and modify data. This involves putting role-based access controls and multi-factor authentication into practice.
- Regular Security Assessments: Conduct regular security assessments to identify and address vulnerabilities in the AI system. These assessments should include penetration testing, code reviews, and vulnerability scans.
- Data Anonymization: Use data anonymization techniques to protect individual privacy. Anonymized data reduces the risk of sensitive information being exposed while still allowing for valuable insights.
Conclusion
As autonomous industrial AI develops, bias and data privacy become critical ethical issues that must be addressed. Through comprehensive knowledge and mitigation of bias, the adoption of strong data privacy safeguards, and adherence to ethical AI best practices, we can fully leverage the potential of AI while preserving the interests of all parties involved.
Ethical AI is not just a technological challenge but a moral imperative. Fairness, transparency, and privacy should be given top priority so that we may create AI systems that are not only effective and potent but also ethical and accountable.
Let us strive to create a future where autonomous industrial AI serves as a force for good, driving progress and innovation while upholding the highest ethical standards.