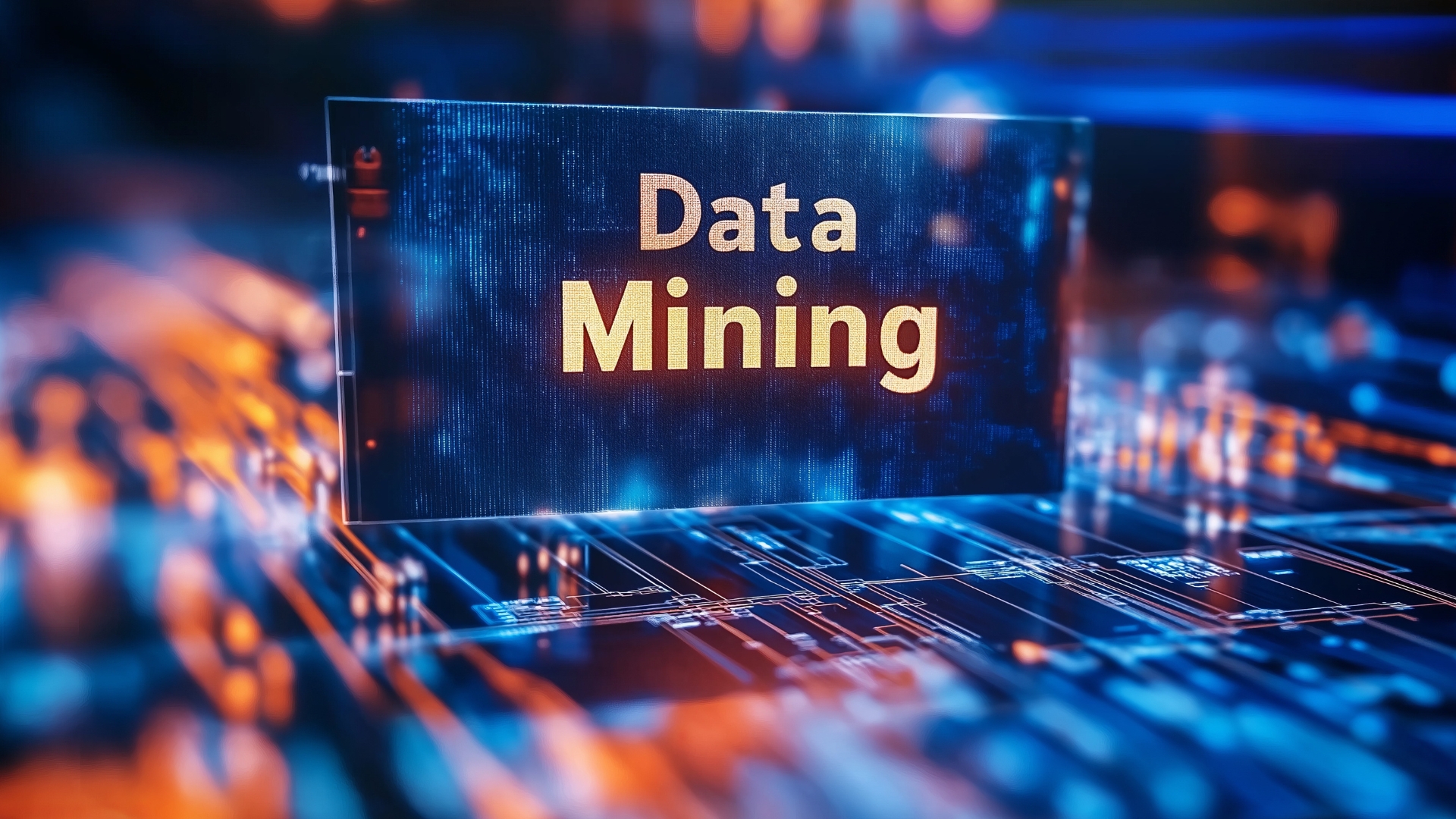
In the ever-evolving tapestry of the digital era, data has emerged as the lifeblood of the modern world. In the world of technical innovation, data mining—once restricted to the occult nooks of academia—has gained prominence.
As vast oceans of data are generated every second, the significance of data mining becomes increasingly apparent. This introductory guide endeavors to peel back the layers of this intricate field, offering an accessible yet thorough exploration of its fundamentals.
Data mining is not merely a byproduct of technological advancement but a response to the overwhelming influx of information. The demand for sophisticated techniques to sort through, evaluate, and extract valuable insights from the jumble of data is growing along with digital ecosystems. The rise of data mining parallels the exponential growth of digital data, marking a pivotal shift in how organizations leverage information.
In today’s interconnected world, data is more valuable than oil. It powers decisions, drives strategies, and shapes the future. The ability to mine data effectively is not just a competitive advantage; it is a necessity. Data mining is altering several industries and how we see the world, including healthcare and corporations.
Explore this article:
- Understanding the Core Concepts
- Types of Data Mining Techniques
- The Process of Data Mining
- Applications of Data Mining
- Tools and Software for Data Mining
- The Future of Data Mining
- Conclusion
Understanding the Core Concepts
What is Data Mining?
The practice of identifying patterns, correlations, and anomalies within huge databases is fundamental to data mining. Finding hidden data that can be turned into insightful knowledge involves using algorithms. While it might sound technical, data mining is essentially about finding the proverbial needle in a haystack, but with the aid of sophisticated tools and techniques.
The Intersection of Data Mining and Big Data
Together, data mining and big data terms that encompass the massive amounts of data produced in today’s digital environment work. Big data provides the raw material, and data mining acts as the sculptor, chipping away to expose important insights. The two have a symbiotic connection. Together, they form the bedrock of modern data analytics.
Types of Data Mining Techniques
Classification: Grouping Data for Clarity
Classification is a technique used to categorize data into predefined classes. Large volumes of data can be more effectively arranged into digestible chunks with this technique, enabling more focused examination. For example, in a business context, classification might be used to segment customers based on purchasing behavior, enabling more personalized marketing strategies.
Clustering: Finding Patterns in Chaos
Unlike classification, clustering does not rely on predefined categories. Instead, it groups data points based on similarities, revealing patterns that might not be immediately apparent. Clustering is often used in market research to identify distinct customer segments or in image analysis to detect similar objects.
Association Rule Learning: Discovering Hidden Relationships
Association rule learning is a technique that uncovers relationships between seemingly unrelated variables within a dataset. A classic example is market basket analysis, where retailers discover that customers who buy bread are also likely to buy butter. This technique is invaluable for cross-selling strategies and improving customer experience.
Regression Analysis: Predicting Future Trends
Regression analysis is a predictive technique that examines the relationship between dependent and independent variables. By understanding these relationships, organizations can forecast future trends and make informed decisions. Regression analysis is used, for instance, in finance to forecast stock values based on historical data.
The Process of Data Mining
Data Collection: The Foundation of Data Mining
The first step in any data mining process is data collection. Data is gathered through various means, such as online platforms (such an example), spreadsheets, or databases. The quality of the data collected directly impacts the success of the data mining process, making this step crucial.
Data Cleaning: Preparing Raw Data for Analysis
Once collected, data often contains errors, duplicates, and inconsistencies that need to be addressed before analysis can proceed. Data cleaning involves identifying and rectifying these issues to ensure that the dataset is accurate and reliable. This step is essential for producing valid and meaningful results.
Data Transformation: Converting Data into Usable Formats
Raw data is often not in a format that is conducive to analysis. Data transformation involves converting this data into a format that can be easily processed by data mining algorithms. This might include normalizing data, encoding categorical variables, or aggregating information.
Data Mining: The Analysis Phase
With the data prepared, the actual mining process begins. This involves applying algorithms to the dataset to extract patterns, correlations, and insights. The choice of algorithm depends on the specific objectives of the analysis, whether it’s predicting future trends, categorizing data, or discovering hidden relationships.
Interpretation and Evaluation: Making Sense of the Results
The final step in the data mining process is interpreting and evaluating the results. This is a prerequisite for the achievement of objective, precise, and beneficial outcomes. The insights derived from data mining must be contextualized within the broader framework of the organization’s goals and objectives.
Applications of Data Mining
Data Mining in Business: Enhancing Decision-Making
One powerful tool used in business to enhance decision-making is data mining. Through consumer data analysis, companies may spot patterns, streamline workflows, and create more potent marketing campaigns. In a market that is evolving constantly, companies that can make data-driven decisions have an advantage over their competitors.
The Role of Data Mining in Healthcare
Data mining is revolutionizing healthcare by enabling personalized treatment plans, predictive analytics, and more precise diagnoses. Healthcare providers can track the evolution of diseases, identify risk factors, and enhance patient outcomes by mining patient data. Data mining is being incorporated into the healthcare sector to enable a more proactive and customized approach to treatment.
Data Mining in Marketing: Understanding Consumer Behavior
Marketers use data mining to gain a deeper understanding of consumer behavior. Identifying the preferences and interests of consumers by analyzing demographic data, social interactions, and purchasing behavior in marketing campaigns. This targeted approach increases the effectiveness of marketing campaigns and enhances customer satisfaction.
The Impact of Data Mining on Social Media Analysis
Data mining is a common practice among social media platforms due to the vast amount of data generated on – or in some cases per day. By analyzing social media data, organizations can gauge public sentiment, monitor brand reputation, and identify emerging trends. This real-time insight is invaluable for shaping marketing strategies and staying ahead of the competition.
Tools and Software for Data Mining
Popular Data Mining Tools in Use Today
Several tools have become synonymous with data mining due to their robust capabilities. Software such as SAS, SPSS, and RapidMiner are among them; they each provide a feature set specific to various facets of data mining. These tools enable analysts to process large datasets, apply algorithms, and generate reports with ease.
Open-Source vs. Proprietary Tools: A Comparative Analysis
The choice between open-source and proprietary tools often depends on the specific needs of the organization. Open-source tools like R and Python offer flexibility and customization at a lower cost, while proprietary tools may provide more comprehensive support and advanced features. Organizations can choose the best instrument for their data mining requirements by being aware of the benefits and drawbacks of each.
The Future of Data Mining
Emerging Trends in Data Mining Technologies
New technologies that promise to expand its capabilities are continually emerging in data mining. Through the application of machine learning, deep learning, and natural language processing, better data mining approaches have been developed. If businesses wish to stay competitive in the data-driven world, they must adapt to these developments.
The Potential of Quantum Computing in Data Mining
The next frontier in data mining is represented by quantum computing. Data mining could be completely changed by quantum computing because of its extraordinary speed at which complex calculations can be processed. Despite being in its infancy, data mining is one of the several uses for quantum computing. These subjects may give rise to fresh insights and growth.
Conclusion
The significance of data mining will only increase as long as we can produce and utilize enormous volumes of data. It is the key to unlocking the insights hidden within the data, driving innovation, and informing decisions. Those who can use data mining to manage the complexity of today’s world will stand to gain in the future.
In a world where data is king, the ability to mine it effectively is more than just a skill; it is a necessity. The future of decision-making across businesses will be shaped by data mining as technologies develop and data becomes increasingly essential to our daily lives. The journey of understanding and mastering data mining is just beginning, and its potential is limitless.